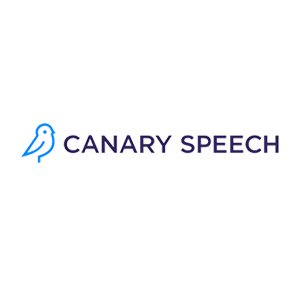
Audio-based Detection of Anxiety and Depression via Vocal Biomarkers
September 28, 2023
This study focuses on mental healthcare, specifically on the detection of anxiety and depression. We present a comparison of results based on the application of various model/feature combinations on the task of detecting anxiety and depression from audio signals of spontaneous speech. The adopted models comprise several different advanced deep neural networks, including CNN, LSTM, and attention networks, and are compared against traditional, shallow machine learning models. Our models are trained based on self-assessment scores: GAD-7 for anxiety and PHQ-8 for depression.
Our best models obtain an unweighted average recall (UAR) of 0.60 for anxiety and 0.63 for the depression task. The result on the anxiety task falls short of the reported self-scored GAD-7 screening reliability of 0.64 just by a small margin and hence shows that this audio-based model can be deployed as an anxiety and depression screening tool. Considering that our models are trained and evaluated on the self-measured, subjective, and hence potentially “noisy” labels, the model performance is highly meaningful and promising towards the goal of automatically and objectively identifying anxiety and depression disorders based on everyday speech, without the time-consuming task of answering the lengthy self-evaluating questionnaires.
Read the Study »